Initiating a Data Strategy from scratch
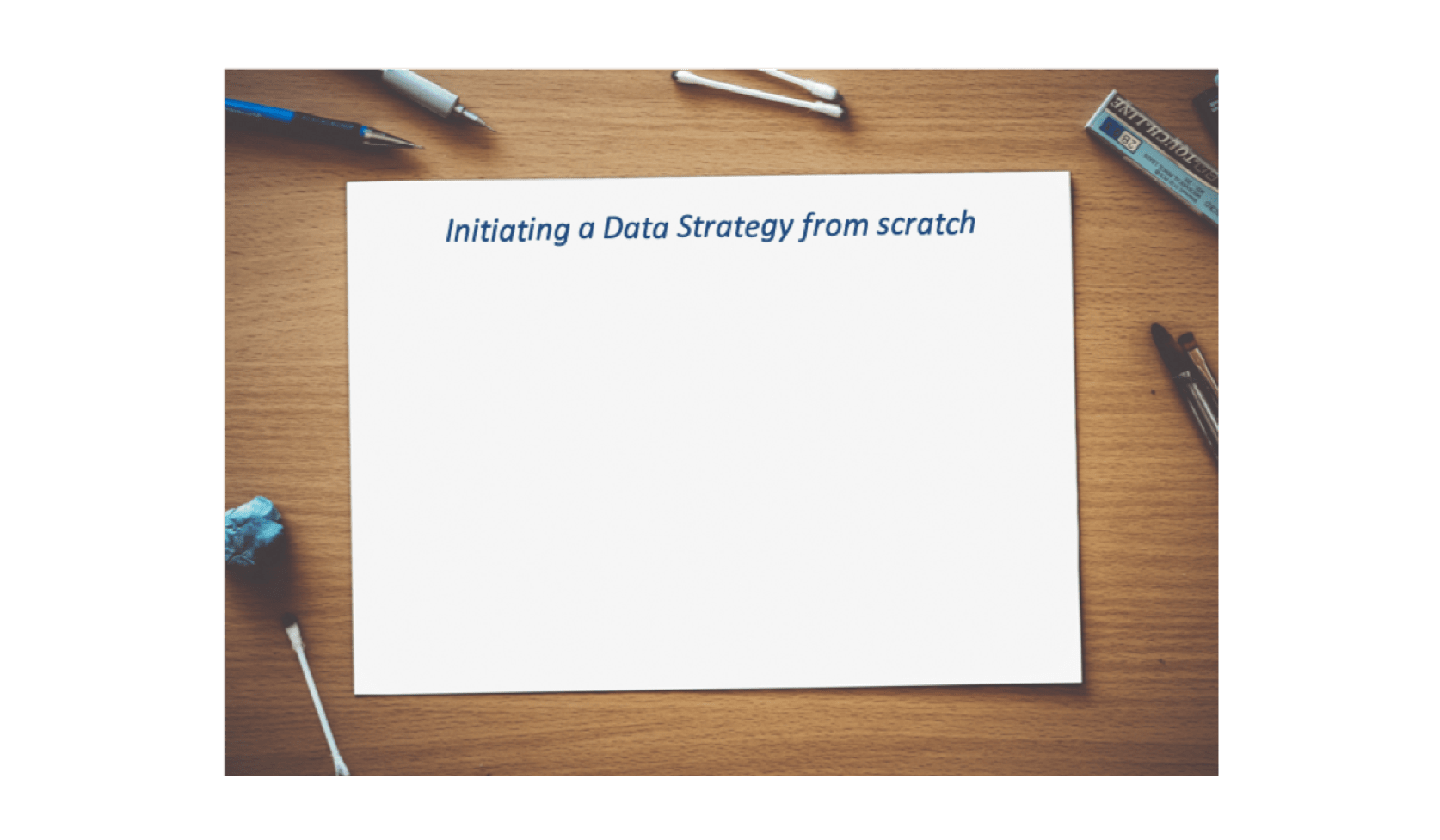
Introduction
Most companies have already identified the leverage of their data assets as a potent growth enabler to efficiently address their daily business pain-points while also increasing innovation speed rate through repeatable approaches. A data strategy primary purpose is above all to support your business strategy by ensuring that your data is managed and used as any other assets hence contributing both to decision-making, operational efficiency optimization or even new value offer creation. Its implementation needs to be steered by a progressive roadmap providing a clear visibility on short, mid and long-term objectives. The underlying question is: how should you initiate your data strategy first-steps?
When designing a data strategy from scratch, many questions arise such as:
- How to start?
- What potential data-driven applications should I target in priority?
- What should be my ideation perimeter?
- How to successfully onboard business teams?
- How should I prioritize initiatives?
This article aims to provide Chief Data Officers (CDO) with some generic hints to address all above issues when initiating a data transformation.
Define explicitly the role you want data to play within your company
Let’s begin with an obvious (but unavoidable) statement: the first brick towards the building of a data strategy is to define your ambition concerning data and AI…
In order to do so, as a first step, you should make an assessment on your current company data maturity (.ie what are your inner competencies, how does the competition leverage its own data, what is your current knowledge about your data patrimony, is there any tool dedicated to data centralization and sharing, etc.). You will indeed require objective insights on your current situation to fully grasp your starting environment before aiming for a workable and realistic company goal.
The second step is to state your ambition concerning data and how it can efficiently support your business strategy. If you’re set on an aggressive market strategy, maybe you would want to secure your competitive “unfair” advantages (.ie a pharmaceutical company with a very strong R&D capitalization aiming to speed even further drug design thanks to AI recommendation) or maybe you would rather grow even more your market share by developing new services (.ie the same pharmaceutical company deciding to monetize its R&D data concerning trials results). On the other hand, if your current strategy is more a defensive one, you could be interested in optimizing overall production costs (.ie the same pharmaceutical company wanting to focus on highest-margin drugs development only would require a clear overview of its production cost main drivers before leveraging them effectively). Having a clear idea of what value you’re expecting from data is a strong driver for your data transformation as explicit goals can easily be shared across your company ecosystem and also contribute to determine your prioritization criteria.
Identify accordingly who should be your data ambition enforcer
Once you got your current data maturity analysis and your target ambition goal, you’re actually able to quantitatively assess the gap between the two of them. You will need to appoint a CDO to carry your transformation from the beginning until his role become later to run and source new data applications.
One of data transformation primary purpose being the erasing of internal barriers between business units through data centralization and sharing, you will need to position your CDO accordingly to your previously expressed ambition. Let’s illustrate this by taking the example of an industrial company wherein Supply Chain leaders and Sales leaders encounter often difficulty to align during the S&OP process: one speak of volumes whereas the other speak of turnover. If the CDO role is to reconcile the two visions, he will require legitimacy from the executive levels to enforce a new vision linking the two others with a pricing notion (which can actually be an operational challenge far more tricky than one might expect). Databases cleaning and data flows implementation will obviously need some human time from both business unit. Jumping locks between business silos isn’t an easy task and requires organizational empowerment to convince target stakeholders…
Start experimenting early on a first concrete application covering the whole AI use-case lifecycle from ideation to industrialization
Our conviction at Quantmetry, is that your data strategy will require to be driven early by concrete operational approaches supporting a first initiative. Indeed, a practical demonstration is the best way to demonstrate the value of data-driven application while also justifying the additional effort provided by your internal teams (interviews realized during the project scoping, data quality efforts, etc.)
Moreover, this first initiative purpose is also to serve as a future internal reference by deploying some first structuring “operational” bricks which will ensure the whole approach repeatability. These “operational” bricks encompass both technical pre-requisites such as an AI-dedicated development platform and organizational pre-requisites such as your data entity structure (see our related article, in French), data governance principles (see our related article, in French), overall processes to steer a data project from ideation to industrialization, etc. Since there is no absolute company-generic guideline to deploy AI projects, many issues will progressively arise depending on the project step. This first project will be the opportunity to spot them first before addressing them to adjust your processes based on operational daily “reality”.
See below our recapitulative analysis grid of what should be the main pillars supporting a data strategy:
Ideation first perimeter should be adapted to your company business strategy and therefore to your previously defined ambition
When initiating a data strategy, a strong challenge lies on settling a “data mindset” within your company so the data strategy can be perennial. This involves an acculturation effort toward your business teams to explain what AI is, how it works and what it could provide them. Restraining your ideation perimeter to your core business pillars is usually a good idea as they directly contribute to the company strategy. This restriction will ease inner communication to explain both AI applications purpose and inner working. Moreover, if you haven’t already mapped your data patrimony with a data cartography (see our related article, in French), it will also be time to start this initiative on the delimited perimeter to support the future ideation process. We often recommend associating at the very least two different business departments into the ideation process to envision potential synergies and address common pain-points. This recommendation is of course an ideal selection scenario and it will have to take into account operational reality such as people and data concrete availability.
Create a use-case portfolio in collaboration with your pre-selected business teams
Building a use-case portfolio is one of the keystones of your data strategy since it will directly fuel its associated roadmap. In order to realize it, ask your previously selected business teams about their daily pain-points and use their acquired field-expertise combined to data science expertise to imagine together data leveraged solutions. The idea is to gather all propositions with a “Christmas wish list” mindset. This ideation process is a key-success factor in your data strategy approach because it will contribute to create both expectations and engagement among your organization. People expressing their business pain-points will indeed be de facto your first identified target customers as an AI provider! To anticipate potential frustration issued from non-selected use-cases during your first iterations, take the time to formalize each idea into your portfolio list in order to value the whole collaborative work achieved and not to give the impression that some propositions were left “aside”. They always could be addressed in the future since they figure explicitly into your portfolio.
Be transparent on your prioritization system criteria
Having a list of many potential use-cases is great, but at some point, prioritization arbitration becomes unavoidable. Because you’re going to raise expectations among your teams, you need to be fully transparent with them about your prioritization criteria upfront of the ideation process. Selection criteria should mix both quantitative and qualitative assessments to be adapted to your company stakes. Qualitative criteria will require a collective agreement from your business experts while quantitative criteria will be assessed through data science prism. The point is to ensure that every stakeholder will be aligned with your future roadmap and feel included in your data strategy objectives even if you won’t address their issue through your first initiative.
Depending on your forecasted budget, you will have a given capacity to ensure a given number of use cases. Do not focus only on “quick-win” projects, even if they are of course very useful to enhance visibility and quickly convince about AI value, synergies between departments should also be considered early. Your synergies analysis needs to be performed on two-axis:
- from a functional business point of view (e.g. a customer knowledge project which contribute to both Marketing/Sales team, which want to know the best offer, and Finance teams, which want to forecast yearly turnover with multiple scenarios)
- from an algorithmic point of view (e.g. selecting a first NLP “easy” use-case which could contribute later to a “tough” one thanks to your data scientists learning curve)
Keep in mind that all these prioritization criteria (business, technical, synergies, etc.) primary purpose is to achieve a relative scoring within your use case portfolio, a ranking enforced by a collective agreement from your department leaders.
Be ready to adopt an agile mindset with a test & learn dynamic approach
Designing and implementing new AI services is no “hard science” since it needs to be adapted to your company specific context including business and IT teams respective working approaches.
What matters is to clearly define mandatory structuring principles to support your data strategy such as your AI department structure, your use-case roadmap, and data governance guidelines without forgetting required data science tools and development platform.
Once this baseline is defined, each project will bring its own lot of issues to tackle such as legal compliance, SLAs definition, data owner identification process on a new data perimeter, data quality alerts reporting, etc. The key is to adopt a test & learn approach and most of all capitalize on successive provided answers in order to build a perennial data strategy.
Conclusion
Initiating a data-driven transformation requires a strategy to define both its objectives and its associated operational roadmap in order to be more than inspirational written concepts about AI added value.
Knowledge of available data should steer the obtention of a prioritized use-case portfolio supporting your core-business strategy and acting as your cornerstone. Indeed, this use-case list will also drive any subsequent additional internal effort (e.g. data quality pre-requisites) within the operational model bricks (organization, processes, resources and technical platform) to deploy, as a second step, since results enhancements would be directly visible and quantifiable to all stakeholders.
Thanks to the cloud technology, experimentations have become affordable for everyone without critical financial backlash in case of failure. Don’t miss the opportunity to try and learn until you gain enough experience to deploy (and maintain) applications at-scale before your rivals do !